Author: Sebastian Wittor
Project Manager Medical Engineering at BAYOOMED
Co-authors: Yussuf Kassem, Christian Riha
Software Engineers at BAYOOMED
In recent years, we have seen a remarkable development in the field of artificial intelligence, particularly in AI Language Models. These advanced systems, most notably Large Language Models (LLMs), have changed the way we interact with technology. These advanced systems open up completely new possibilities in research, education, creativity and problem solving. Why are LLMs so relevant? With the ever-growing and increasingly important need for natural and seamless human-machine interactions, Large Language Models have become an indispensable part of modern technologies. They allow complex tasks to be accomplished, innovative solutions to be found and new ways of interacting to be created. In this blog post, we take a deep dive into the world of LLMs. We explain how they work, provide an overview of the different types and highlight their versatile applications.
What are Large Language Models?
Large Language Models (LLMs) are highly developed AI systems that are capable of precisely understanding, generating and efficiently processing human language in all its many facets. These impressive models are based on advanced neural networks. They use powerful deep learning techniques to continuously learn from huge and diverse text datasets. In contrast to older, purely rule-based systems, which relied on rigid and often limited linguistic structures, LLMs acquire their knowledge directly and flexibly from the underlying data. This enables them to accurately capture context-dependent meanings, easily understand idiomatic and colloquial expressions and even generate creative texts that in many cases can hardly be distinguished from human texts.
The versatile possibilities of Large Language Models:
A key advantage of LLMs is their ability to process natural language dynamically and flexibly. This clearly surpasses classic approaches such as rule-based systems and conventional machine learning algorithms. Although rule-based systems are deterministic and transparent, they quickly reach their limits when it comes to processing natural language and complex contexts. The revolutionary ability of large language models to understand and apply language at a near-human level is fundamentally changing how we use information and interact with technology.
How do LLMs work?
The way large language models (LLMs) work is as impressive as it is complex. At its core is the principle of “unsupervised learning”, in which the model independently recognizes patterns and structures from huge text data – without direct human guidance. This learning process takes place in two central phases.
Training phase
The first step is to carefully collect enormous amounts of text data from a variety of different sources. These sources can include books, scientific articles, websites or social media posts. The quality and variety of this data plays a crucial role, as it significantly influences the performance and versatility of the resulting model.
The collected data is first carefully cleansed and then converted into a format that is understandable for the model. This process often involves tokenization, in which the text is broken down into smaller units known as tokens. Tokenization can take place at word, subword or even character level. Each of these methods has specific advantages and disadvantages and is suitable in different ways depending on the application.
Most modern Large Language Models (LLMs) are based on the so-called “Transformer” architecture, which was developed and presented by Google researchers in 2017. This pioneering architecture enables the model to precisely capture the context of words and sentences over long distances and thus develop a deeper understanding of complex linguistic contexts. Core elements of the Transformer architecture are:
- Self-attention mechanisms: Enable the model to understand the relationships between different words in a sentence.
- Multi-Head Attention: Allows the model to consider different aspects of the context simultaneously.
- Feed-forward networks: Process the information from the attention layers further.
During training, the model is presented with a large amount of text data, which it analyzes and processes. In doing so, it learns to recognize patterns and relationships in the language by continuously trying to predict the next word or token in a given sequence. This process, often referred to as “masked language modeling”, enables the model to develop a deep understanding of language structures, grammatical rules and contextual meanings.
After the initial training, the model is often specialized for specific tasks or domains in order to expand its application possibilities in a targeted manner. This process takes place through further training with carefully selected, specific data sets. This so-called fine-tuning makes it possible to significantly increase the model’s performance for specific applications while fully preserving its general language capabilities and versatility.
In this phase, the model learns to understand instructions precisely and follow them effectively. This is crucial for the model’s ability to respond appropriately and contextually to user requests. Instruction tuning enables large language models (LLMs) to respond flexibly to a variety of different tasks without the need for separate training for each individual task.
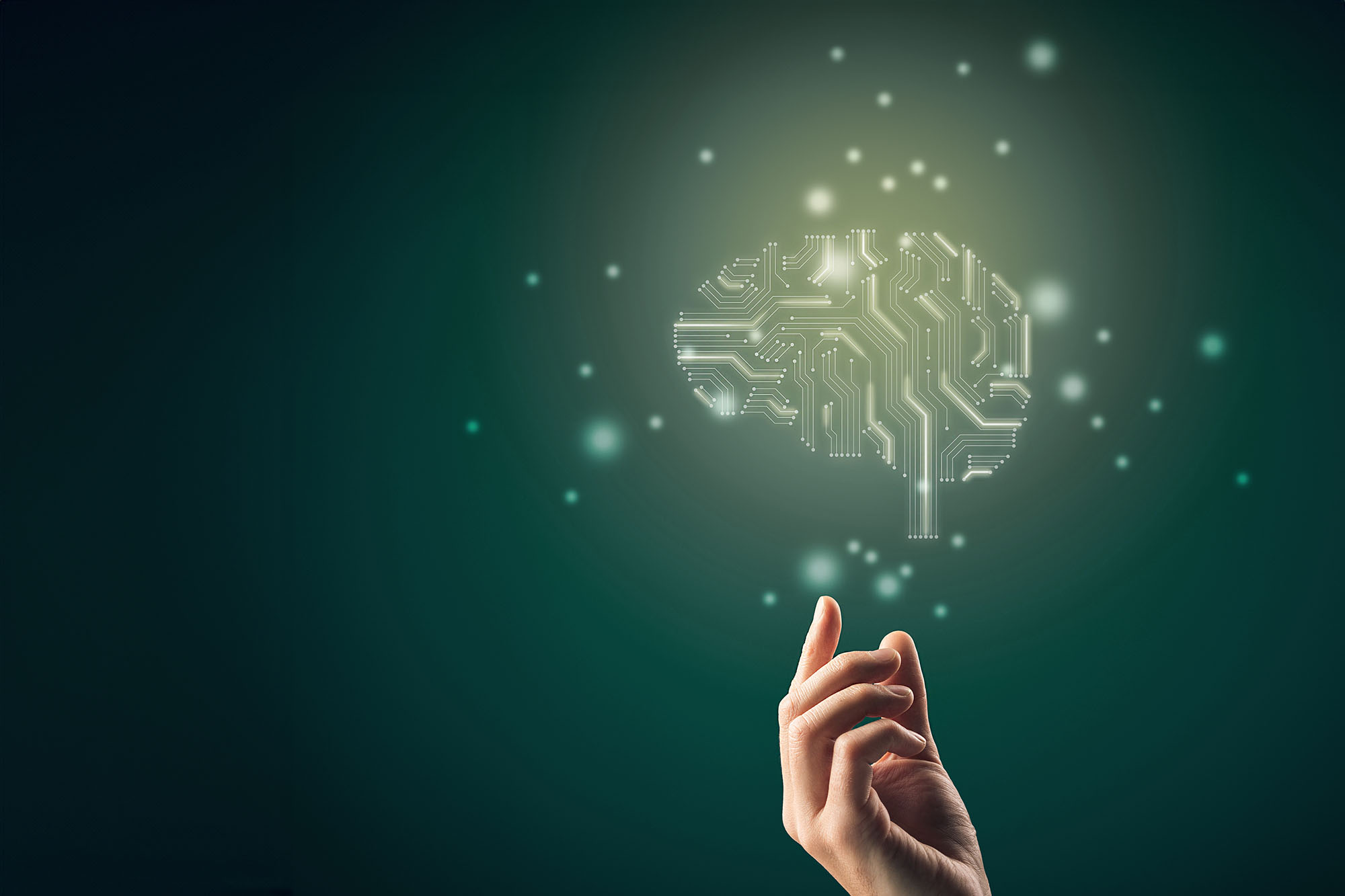
Inference phase
When a trained LLM receives a request, it goes through the following steps:
The power of LLMs lies in their ability to perform these steps with astonishing speed and precision, often in fractions of a second. This enables real-time applications such as chatbots, translation services and interactive assistance systems.
The input is first tokenized and then converted into a format that the model can understand. The same tokenization scheme that was already used during training is often applied to ensure consistent and correct processing.
The model analyzes the context of the input by drawing on the patterns and structures learned during training. The self-attention mechanisms are used specifically to precisely recognize and understand the relationships and dependencies between different parts of the input.
Based on the context of the input and the patterns learned during training, the model generates a suitable response step by step. This process is often done word by word or token by token. At each individual step, the model carefully calculates the probabilities for the next possible token and selects the most likely matching token based on this.
The generated response is then converted into clearly readable text and presented to the user. This process may include additional steps such as detokenization or special formatting to ensure that the output is both correct and easy to understand and use.